Why are Data Scientists adored despite their rarity?
"From the birth of humanity till 2003, 5 exabytes of information were created, but that much information is being created every two days now."
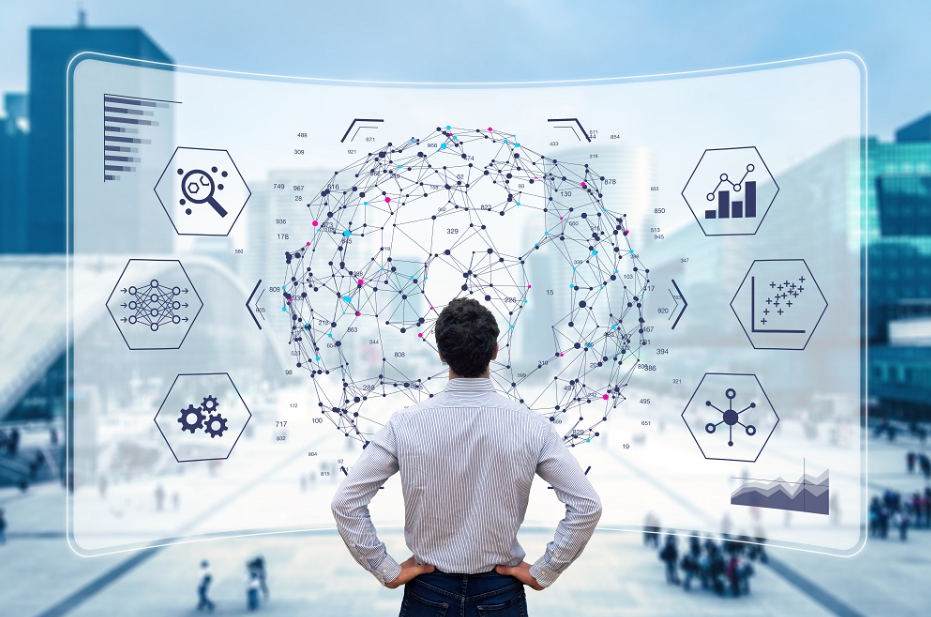
One of the most in-demand professions of the twenty-first century is data science. It has evolved into a buzzword that is used frequently these days. For the purposes of modelling, data mining, and research, a data scientist is in charge of establishing and developing the procedures and organizational structures for complicated, large-scale data sets.
Data scientists use their programming, statistical, mathematical, and analytical talents to gather, examine, and understand vast amounts of data. He is the one who provides insights from evaluating corporate data to the product, sales, and marketing departments.
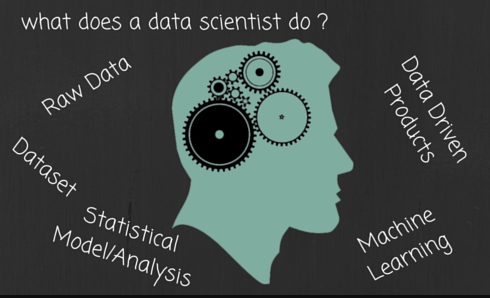
The following are the daily responsibilities of a Data Scientist:
He must extract big data sets that have been gathered from numerous internal and external sources. The information that is gathered may be structured or unstructured, but it must be set up in a way that makes it simple to comprehend and use.
The main objective is to thoroughly clean the data, remove the errors, and reject the useless data. He finds any missing values that may be present in the data sets.
The process of extracting and evaluating data from a firm database aid in improving product development, marketing initiatives, and business expansion plans.
Creating open-ended research questions and researching numerous subjects. To properly construct a report based on the research, the following questions must be addressed.
Predicts business issues and identifies solutions to address them. In order to identify patterns and opportunities, he also studies and analyses the data.
With the aid of machine learning and statistical techniques, prepares the data and employs predictive modelling to improve and maximise customer experiences, revenue generation, and other business outcomes.
Uses data visualisations and reports to convey the conclusions and solutions to the management and IT departments.
Develops new algorithms to use on data sets in order to tackle complex problems, as well as new tools and methods to automate the task.
Oversees creating procedures and equipment to track, examine, and assess the performance of models and the reliability/accuracy of data.
Collaborates with the various functional teams to put the models into practise and keep track of the results.
Tools used to do the tasks
Without any tools, it is impossible to complete these activities. The most crucial tools a data scientist requires are:
1. Programming Languages (Python/R):
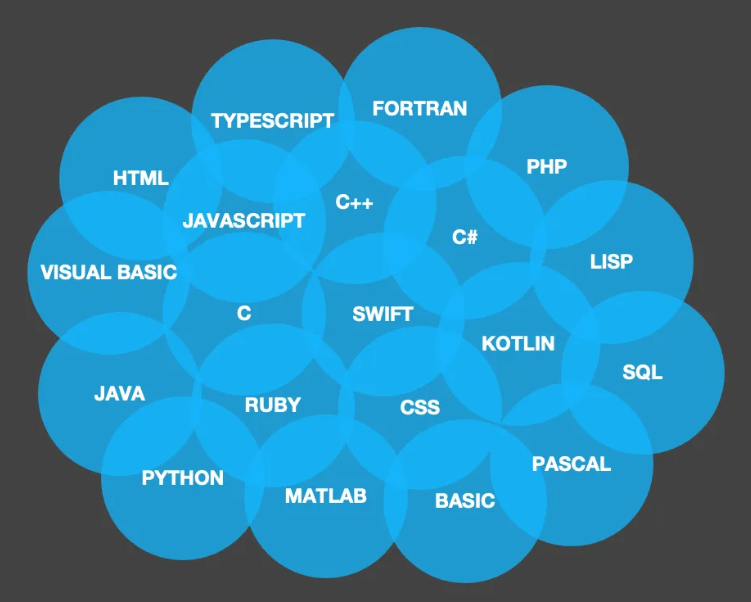
Programming is the use of a set of written instructions to direct a computational device (like a computer) to carry out a series of activities, changing a function inside the machine. Depending on every programming language the engineer picks, a separate set of words and instructions are examined. This is because each programming language has its own syntax and grammar. Additionally, a compiler converts this written code into information for a computer. The way a computer or computing device understands the syntax differs per language.
Python and R are among the most in-demand programming languages for data scientists. Programming languages are among the most useful software tools for data scientists to understand, while some people working with machine learning and statistics may also find having knowledge of black-box technologies useful. Python, being the most popular language of this year, is becoming the first choice of data scientists.
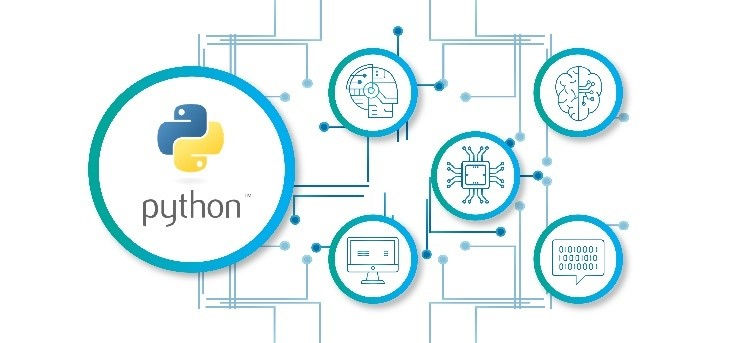
Python is a free, general-purpose programming language. It contains a wide range of libraries, including some of the best artificial intelligence and machine learning tools. Python is a fantastic choice for a data scientist undertaking extensive data cleaning, machine learning and artificial intelligence because it is especially well-suited for beginners.
2. Structured Query Language (SQL):
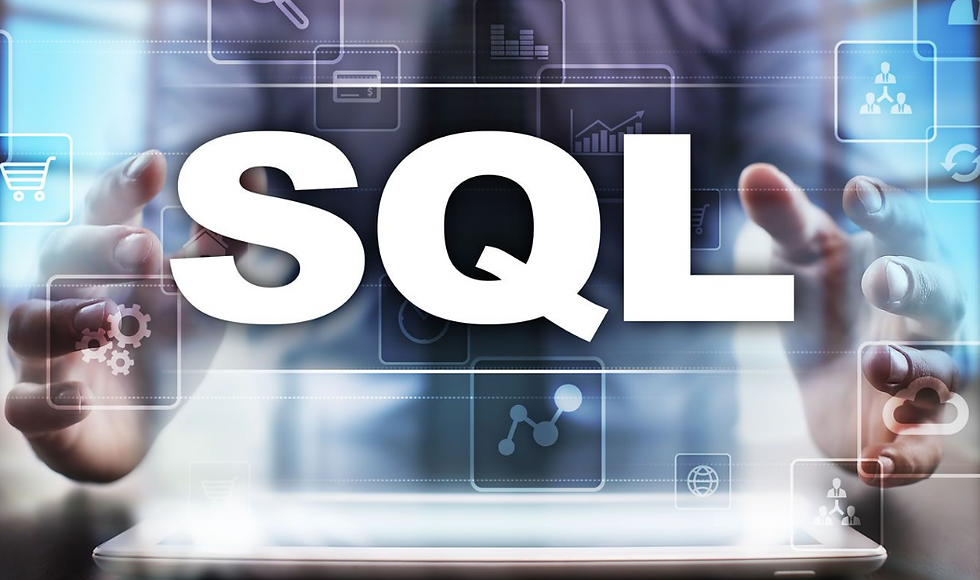
Structured Query Language, or SQL, is a widely used query language for maintaining and querying relational databases. It enables the creation, upkeep, and retrieval of data from relational databases. Through a variety of straightforward statements, SQL enables you to insert, update, remove, change, and retrieve data.
Its widespread use can be attributed to how simple it is to use and comprehend. As a result, the syntax employed in SQL is extremely close to English words.
SQL databases come in many forms, including SQLite, MySQL, Oracle, Microsoft SQL Server, and others. Depending on the needs of the data, each of them works better in certain situations.
You may efficiently examine and display your dataset with SQL for Data Science to generate reliable results. It will assist you in dealing with outliers, missing and null values, and other data abnormalities.
The usage of SQL for data science is becoming the norm across many of the industry titans, like Facebook, Google, Amazon, Netflix, Uber, etc. Each of the aforementioned uses SQL to carry out different Data Science operations.
The popular programming languages R and Python were outperformed by SQL for Data Science in the 2017 and 2018 StackOverflow Developer Survey.
3. Microsoft EXCEL:
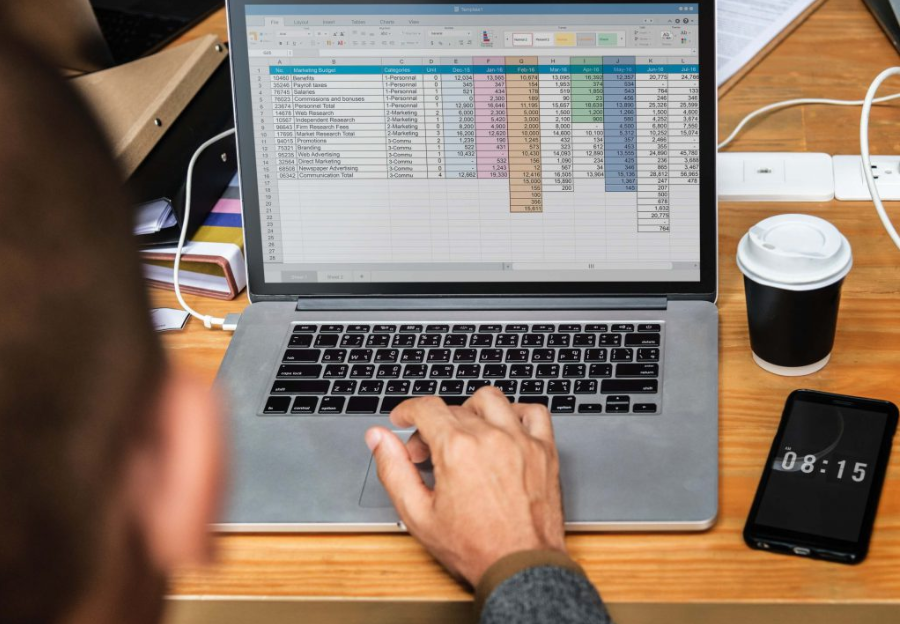
Microsoft Excel is a spreadsheet application that was developed in 1985 and can be used to arrange information and data into rows and columns. Excel can be used for data entry and simple data presentation, much like database management systems. Excel can be used to visualize data recorded in a spreadsheet by creating charts and graphs as well as performing mathematical and statistical calculations. Learning how to use Excel can make managing information and data easier and more effective for anyone utilises it thanks to its variety of functions and formulae.
The simplicity of usage and widespread accessibility of Microsoft Excel make it a good introductory tool for both students and professionals, especially for beginning data scientists.
Before a data science project begins it is important to perform an exploratory analysis in order to learn more about any potential findings within the dataset. Microsoft Excel has multiple functions programmed into the software which makes it simple to explore a dataset through sorting, filtering, and pivot tables.
4. BI Tools (Power BI/Tableau):
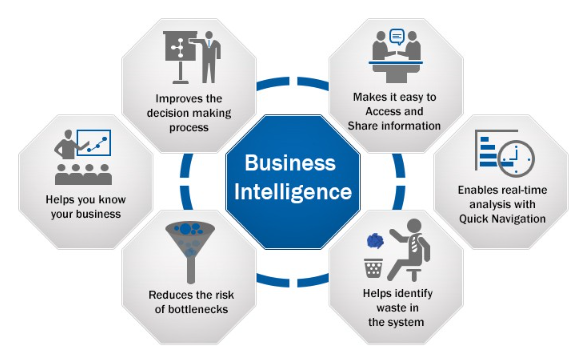
Your dashboards and visualizations might not have the same effect without a story, an explanation, and context. Each observer may interpret the meaning differently if all you have is a visualization. Data scientists need to give the data a voice (or other analytics users).
The tale must be told, followed by an explanation of the findings, such as an outlier that is skewing a trend. After that, your audience can act in a reasoned manner since action requires context. Using data to guide decision-making is, in a broad sense, the goal of using a BI tool.
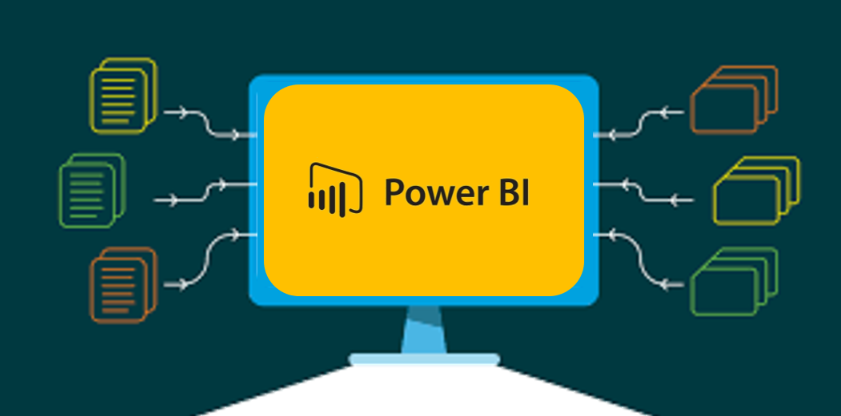
Power BI is the BI tool that is in the demand. For the data analytics and data science, Power-Bi is an all-in-one, advanced tool. It can be compared to a high-level application more related to Microsoft Excel than a programming language-type application. Even though there is occasionally programming required, most end users could probably get by with a little experience and very little dedication.
Conclusion: Depending on the role you choose to play, there are numerous employment choices in the large subject of data science. Which are:
Data Architect
Data Engineer
Data Science Manager
Statistician
Machine Learning Engineer
Decision Scientist
Join IOTA ACADEMY today to become a certified Data Scientist and grow your career.
Thanks for reading...
The best institute for data science, learned by IITians, covers all the material in a fluid manner.
This is what I was looking for, every topic is covered very nicely. Thanks