Common Data Analysis Mistakes and How to Avoid Them
- IOTA ACADEMY
- Feb 27
- 4 min read
Updated: Mar 25
Improve your data analysis skills by avoiding common mistakes. Ensure accuracy, effectiveness, and better decision-making with expert insights
Inadequate Clear Objectives
Unclear objectives are one of the common pitfalls in data analysis. You should know what you want to get before starting to work with data. Without clear objectives, it will be difficult to find anything even in a vast amount of data; your analysis may end up being purposeless and have the wrong conclusion. Whether you have customer trends or predict future sales, define the objective.
How to Avoid It: Define the question or problem you are investigating. Clearly, define what results you expect the analysis to achieve. A defined objective helps guide the choice of tools and techniques.
Lack of Proper Data Quality
The second mistake is that the quality of the data that is being analyzed is not considered. Poor-quality data can lead to inaccurate conclusions. Missing values, duplicate entries, or irrelevant data can distort the results. It is very important to clean the data before starting the analysis.
How to Avoid It: Check for missing or inconsistent data regularly. Use data cleaning techniques like imputation or removing duplicates. A good data analytics course in Indore will teach you how to deal with and clean data properly.
Overlook Data Visualization
Data representation is important in the interpretation of complex data. Even with the best available data, the trends or patterns can easily be missed without proper visualization. Mere data and tables may confuse or mislead the decision-making process.
How to Avoid It: Use charts, graphs, and dashboards for data visualization. Proper tools help make insights more talkable. How to use visualization techniques can be seen in many of the sections of offline data analysis courses.
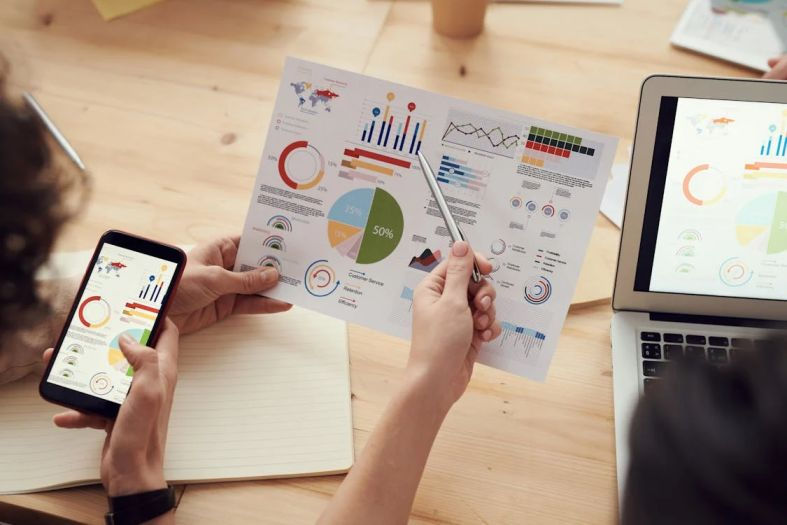
Relying Too Much on Statistical Significance
Many statistics analysts over-rely on statistical significance. The fact that a result is statistically significant does not necessarily mean it is practically relevant. Statistical significance tests can sometimes produce false positives, leading to incorrect conclusions.
How to Avoid It: Look at the context and relevance of your findings. In addition to looking at statistical significance, focus on practical significance. Also, focus on the business context when interpreting your results.
Not Accounting for Bias
Bias in data analysis may yield skewed results. This bias may emanate from the process of collecting data, sampling methods, or even the analysts involved. Ignoring these biases can lead to incorrect conclusions that may not be acceptable for the whole population.
How to Avoid It: Be always aware of potential biases in your data. Consider using techniques such as random sampling or double-checking your methods. Joining data analyst coaching in Indore can help you identify and address these biases effectively.
Using Inappropriate Tools
Another common error is using the wrong tool for the job. Different data analysis tasks require different tools. Wrong conclusions, inefficient processing, and slow processing times may result if the wrong tool is used.
How to Avoid It: Understand a variety of data analysis tools and software. Each tool is good for one thing but lousy for something else. Enrollment in the best IT courses training in Indore will help understand which tool should be used under what circumstances.
Inadequate Documentation
They try to get over with the analysis in a rush and forget to document their processes. Documentation will help trace the process through which conclusions have been reached. Others may also scrutinize your work to ensure transparency and accuracy.
How to Avoid It: Write down the methods, assumptions, and results. Documentation also helps in debugging and improvements of future analysis.
Misinterpretation of Correlation and Not Causation
This is confusing correlation with causation. The fact that two variables are moving together does not mean one causes the other. Relying on correlation alone can lead to inaccurate conclusions.
How to Avoid It: Always consider whether one variable truly influences the other. Use additional analysis techniques, such as regression testing, to identify causality.
Neglecting the Human Element
Data analysis is not only about numbers and algorithms. Human intuition also plays a role. Analysts need to use their knowledge and experience when making sense of the data. Disregarding the human factor results in poor insight.
How to Avoid It: Combine technical analysis with critical thinking. Data should support, not replace, human decision-making.
Skipping the Review Process
Most data analysts do not review their work, assuming that it is free of errors. Without a second opinion, mistakes can go unnoticed, which may affect decision-making.
How to Avoid It: Always have your analysis reviewed by another analyst or team member. Peer reviews help catch mistakes that might have been overlooked.
Conclusion
These are the common mistakes in data analysis. Avoiding them will make your analysis of higher quality and more accurate. Whether you enrol in a data analyst course offline or already work as an analyst, keeping these errors in mind will improve your skills considerably. Make sure to define clear objectives, clean up your data, use proper tools, and consider biases at every step. In addition, always combine data insights with human judgment for the best result.
If you would like to gain more skills in data analysis, then joining the data analytics course in Indore or getting coaching for a data analyst in Indore can really help you obtain the knowledge you need and be coached.
Commenti